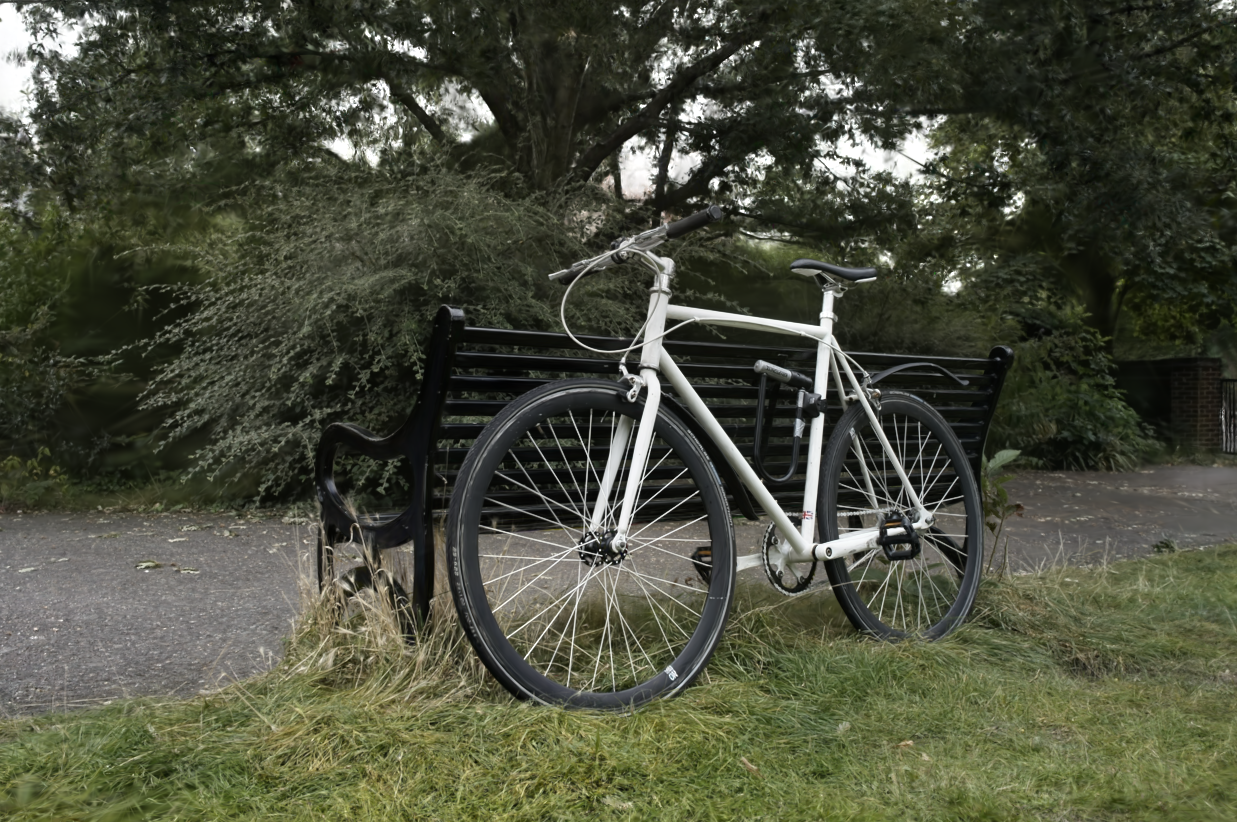
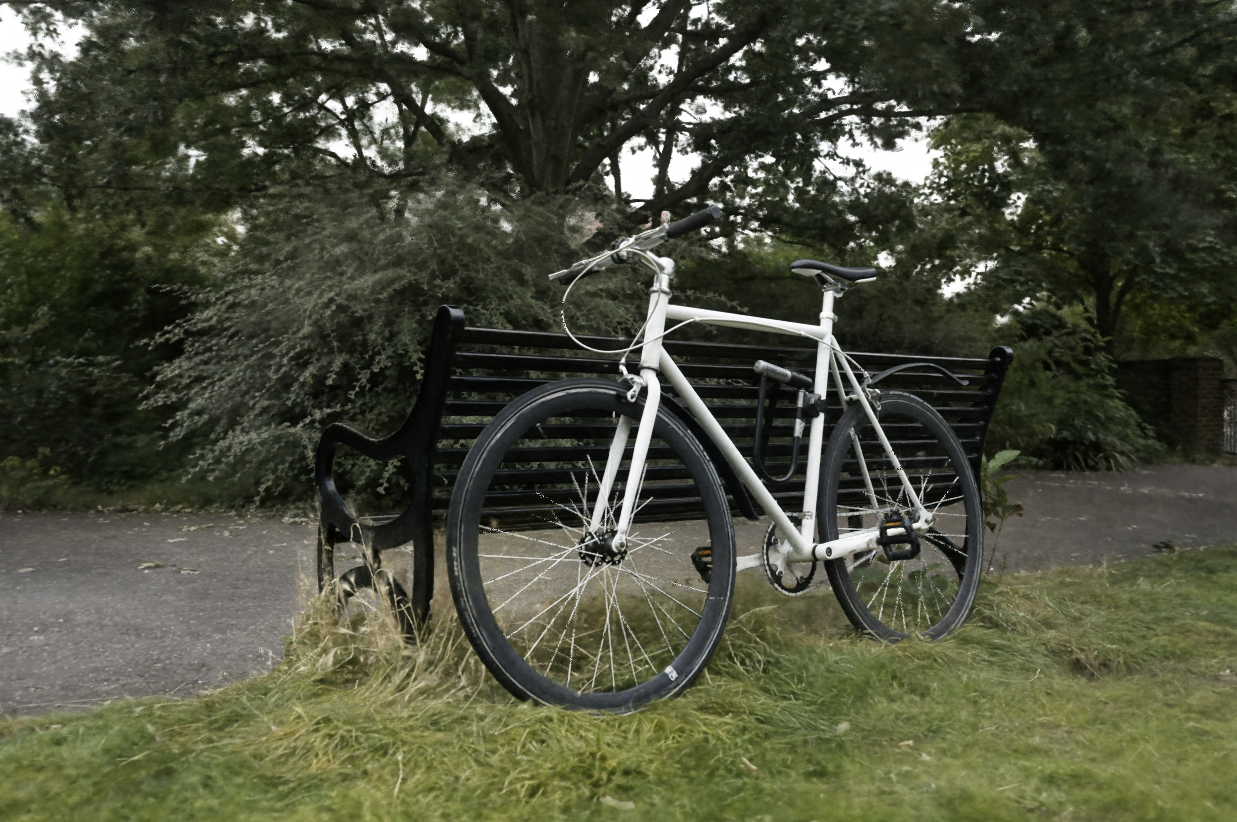
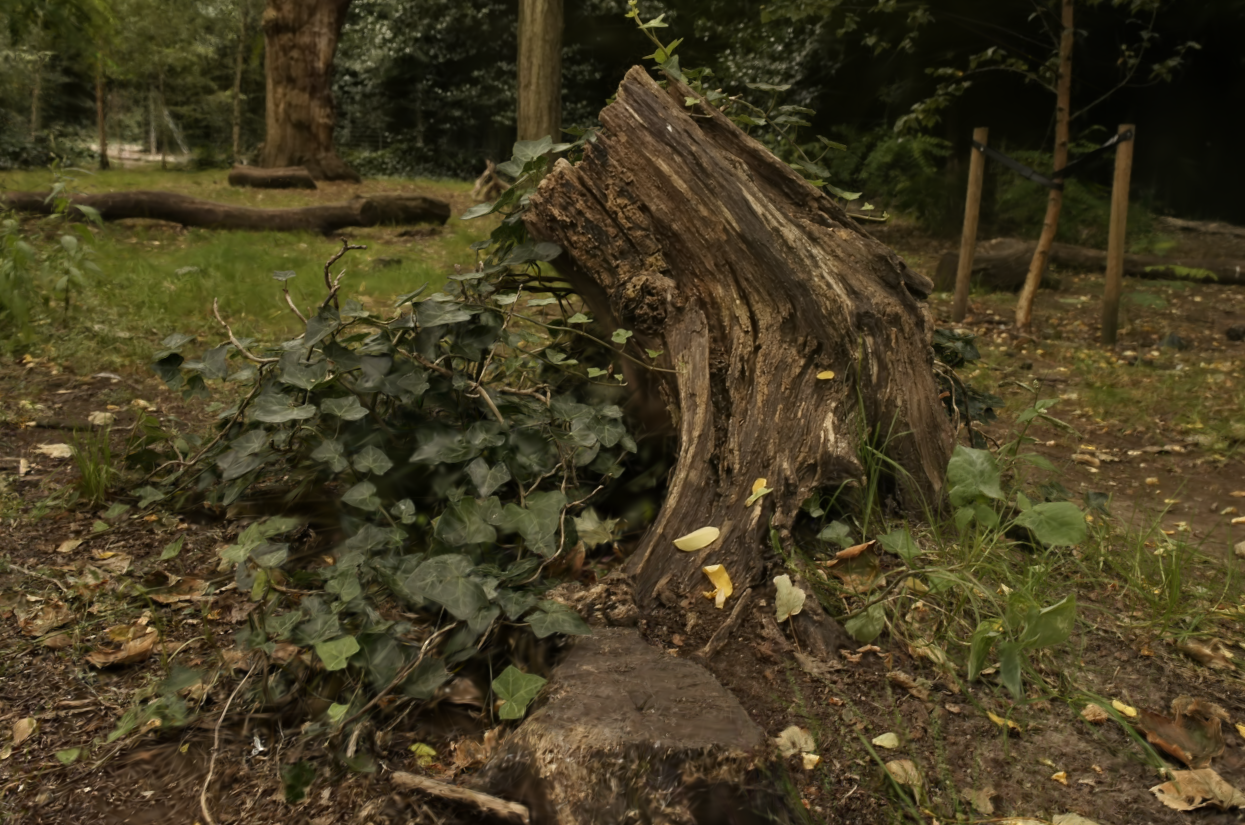
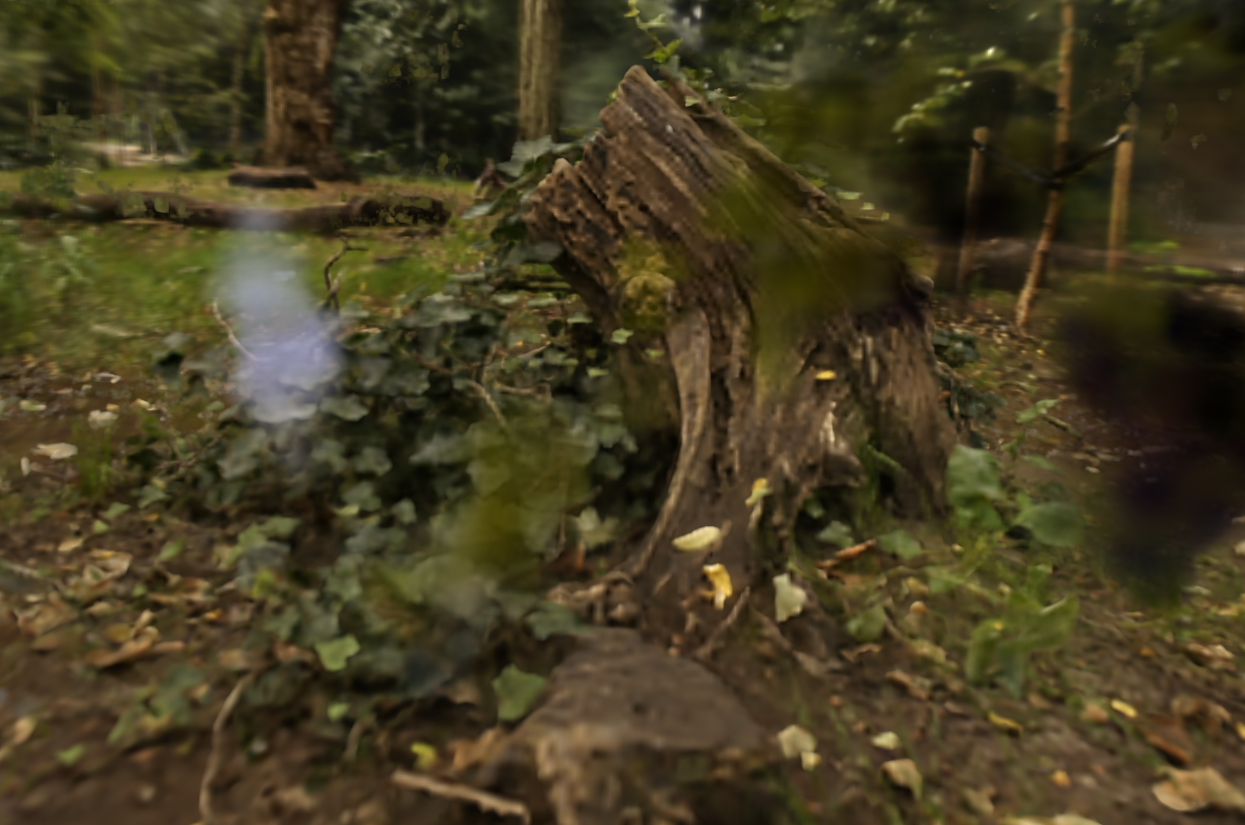
Radiance Field methods have recently revolutionized novel-view synthesis
of scenes captured with multiple photos or videos. However, achieving high
visual quality still requires neural networks that are costly to train and render,
while recent faster methods inevitably trade off speed for quality. For
unbounded and complete scenes (rather than isolated objects) and 1080p
resolution rendering, no current method can achieve real-time display rates.
We introduce three key elements that allow us to achieve state-of-the-art
visual quality while maintaining competitive training times and importantly
allow high-quality real-time (≥ 100 fps) novel-view synthesis at 1080p resolution.
We tested our algorithm on a total of 13 real scenes taken from previously published datasets and the synthetic Blender dataset. In particular, we tested our approach on the full set of scenes presented in Mip-Nerf360 [Barron 2022], which is the current state of the art in NeRF rendering quality, two scenes from the Tanks and Temples dataset [Knapitsch 2017] and two scenes provided by Deep Blending [Hedman 2018]. For the full evaluation please check the paper and the supplemental.
@Article{kerbl3Dgaussians,
author = {Kerbl, Bernhard and Kopanas, Georgios and Leimk{\"u}hler, Thomas and Drettakis, George},
title = {3D Gaussian Splatting for Real-Time Radiance Field Rendering},
journal = {ACM Transactions on Graphics},
number = {4},
volume = {42},
month = {July},
year = {2023},
url = {https://repo-sam.inria.fr/fungraph/3d-gaussian-splatting/}
}
This research was funded by the ERC Advanced grant FUNGRAPH No 788065. The authors are grateful to Adobe for generous donations, the OPAL infrastructure from Université Côte d’Azur and for the HPC resources from GENCI–IDRIS (Grant 2022-AD011013409). The authors thank the anonymous reviewers for their valuable feedback, P. Hedman and A. Tewari for proofreading earlier drafts also T. Müller, A. Yu and S. Fridovich-Keil for helping with the comparisons.
[Müller 2022] Müller, T., Evans, A., Schied, C. and Keller, A., 2022. Instant neural graphics primitives with a multiresolution hash encoding
[Hedman 2018] Hedman, P., Philip, J., Price, T., Frahm, J.M., Drettakis, G. and Brostow, G., 2018. Deep blending for free-viewpoint image-based rendering. ACM Transactions on Graphics (TOG), 37(6), pp.1-15.
[Barron 2022] Barron, Jonathan T., et al. "Mip-nerf 360: Unbounded anti-aliased neural radiance fields." Proceedings of the IEEE/CVF Conference on Computer Vision and Pattern Recognition. 2022.
[Fridovich-Keil and Yu 2022] Fridovich-Keil, Sara, et al. "Plenoxels: Radiance fields without neural networks." Proceedings of the IEEE/CVF Conference on Computer Vision and Pattern Recognition. 2022.
[Knapitsch 2017] Knapitsch, Arno, et al. "Tanks and temples: Benchmarking large-scale scene reconstruction." ACM Transactions on Graphics (ToG) 36.4 (2017): 1-13.