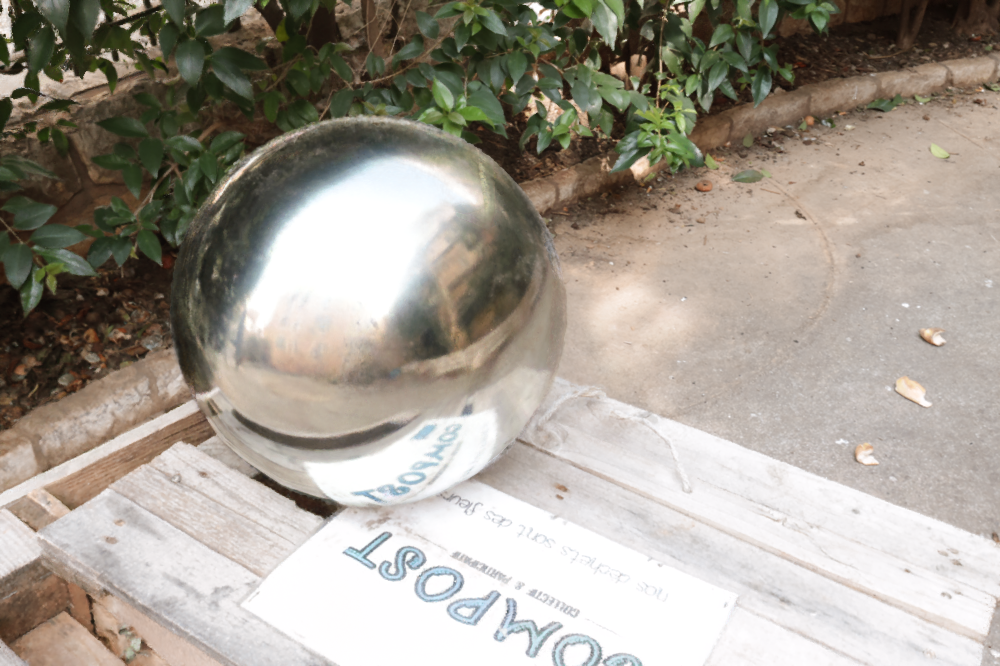
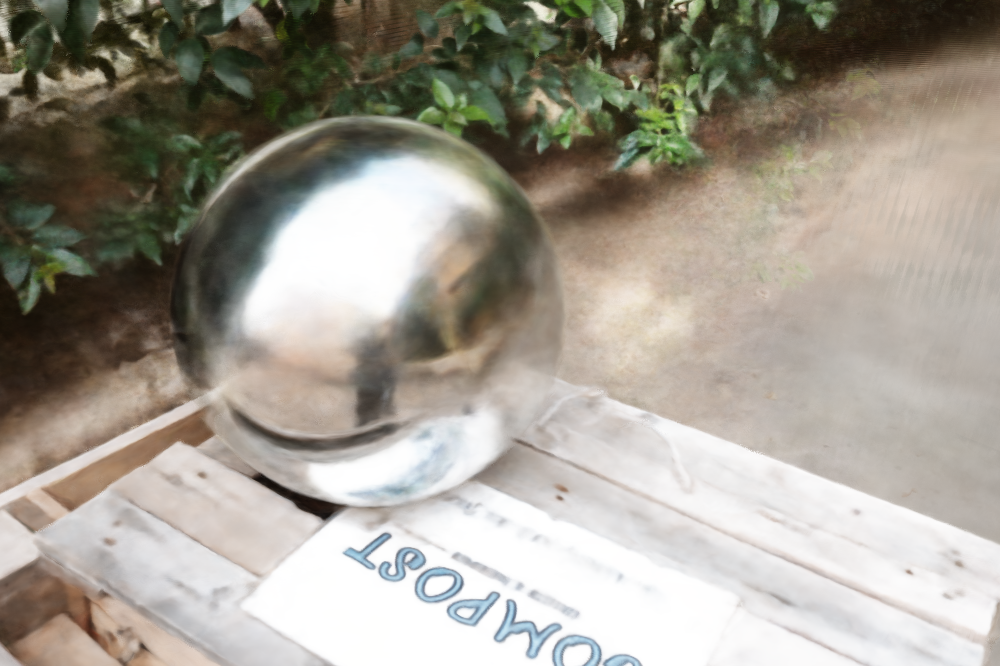
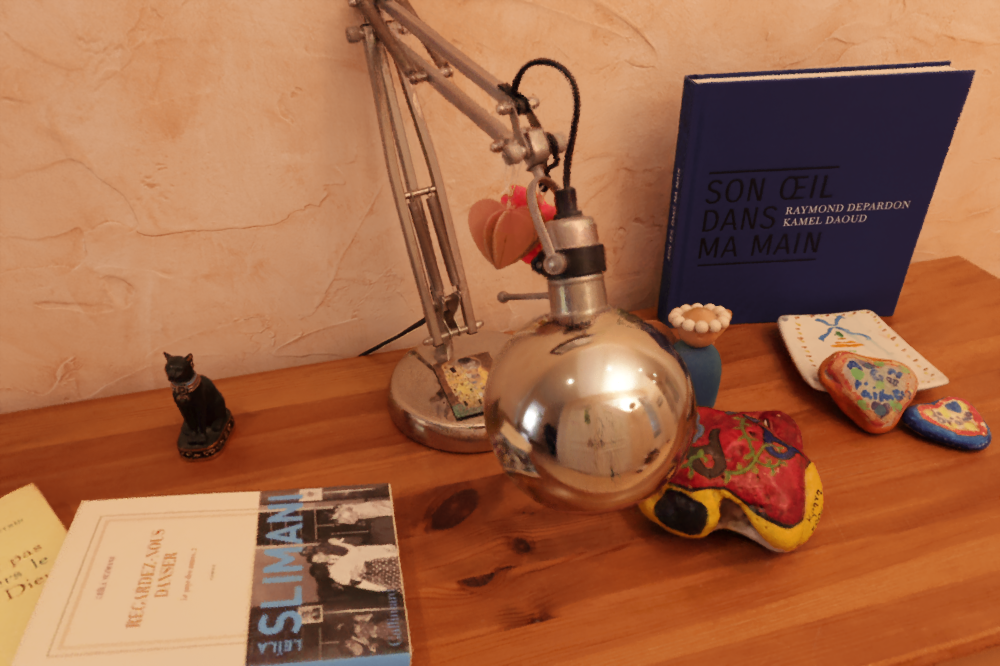
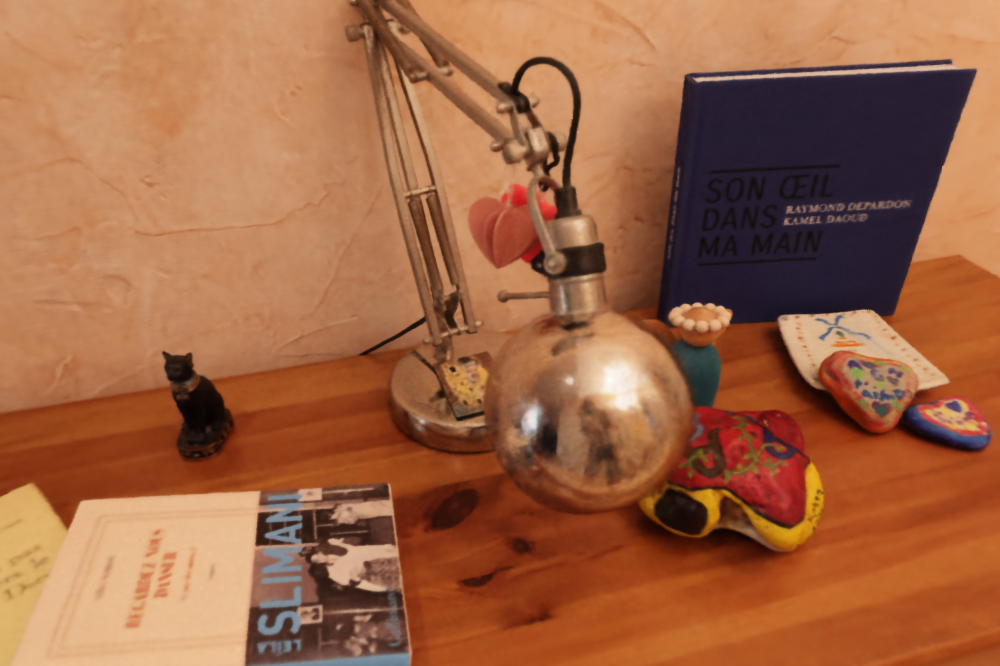
View-dependent effects such as reflections pose a substantial challenge for image-based and neural rendering algorithms. Above all, curved reflectors are particularly hard, as they lead to highly non-linear reflection flows as the camera moves.
We introduce a new point-based representation to compute Neural Point Catacaustics allowing novel-view synthesis of scenes with curved reflectors, from a set of casually-captured input photos. At the core of our method is a neural warp field that models catacaustic trajectories of reflections, so complex specular effects can be rendered using efficient point splatting in conjunction with a neural renderer. One of our key contributions is the explicit representation of reflections with a reflection point cloud which is displaced by the neural warp field, and a primary point cloud which is optimized to represent the rest of the scene. After a short manual annotation step, our approach allows interactive high-quality renderings of novel views with accurate reflection flow.
Additionally, the explicit representation of reflection flow supports several forms of scene manipulation in captured scenes, such as reflection editing, cloning of specular objects, reflection tracking across views, and comfortable stereo viewing.
We compare our method with previous IBR and recent neural rendering methods; the most meaningful comparisons are qualitative visual inspections of the videos. We also provide quantitative comparisons in the paper and an ablation study.
@article{kopanas2022neural,
title={Neural Point Catacaustics for Novel-View Synthesis of Reflections},
author={Kopanas, Georgios and Leimk{\"u}hler, Thomas and Rainer, Gilles and Jambon, Cl{\'e}ment and Drettakis, George},
journal={ACM Transactions on Graphics},
volume={41},
number={6},
pages={Article--201},
year={2022}
}
This research was funded by the ERC Advanced grant FUNGRAPH No 788065 http://fungraph.inria.fr. The authors are grateful to the OPAL infrastructure from Université Côte d’Azur and for the HPC resources from GENCI–IDRIS (Grant 2022-AD011013409). The authors thank the anonymous reviewers for their valuable feedback, P.Hedman for proofreading earlier drafts, T.Louzi for the Silver-Vase object, S.Kousoula for help editing the video and S.Diolatzis for thoughtful discussions.
[Müller 2022] Müller, T., Evans, A., Schied, C. and Keller, A., 2022. Instant neural graphics primitives with a multiresolution hash encoding
[Hedman 2018] Hedman, P., Philip, J., Price, T., Frahm, J.M., Drettakis, G. and Brostow, G., 2018. Deep blending for free-viewpoint image-based rendering. ACM Transactions on Graphics (TOG), 37(6), pp.1-15.
[Kopanas 2021] Kopanas, G., Philip, J., Leimkühler, T. and Drettakis, G., 2021, July. Point‐Based Neural Rendering with Per‐View Optimization. In Computer Graphics Forum (Vol. 40, No. 4, pp. 29-43).
[Barron 2021] Barron, J.T., Mildenhall, B., Tancik, M., Hedman, P., Martin-Brualla, R. and Srinivasan, P.P., 2021. Mip-nerf: A multiscale representation for anti-aliasing neural radiance fields. In Proceedings of the IEEE/CVF International Conference on Computer Vision (pp. 5855-5864).